Person Griddying in a Science Lab AI: Building Success
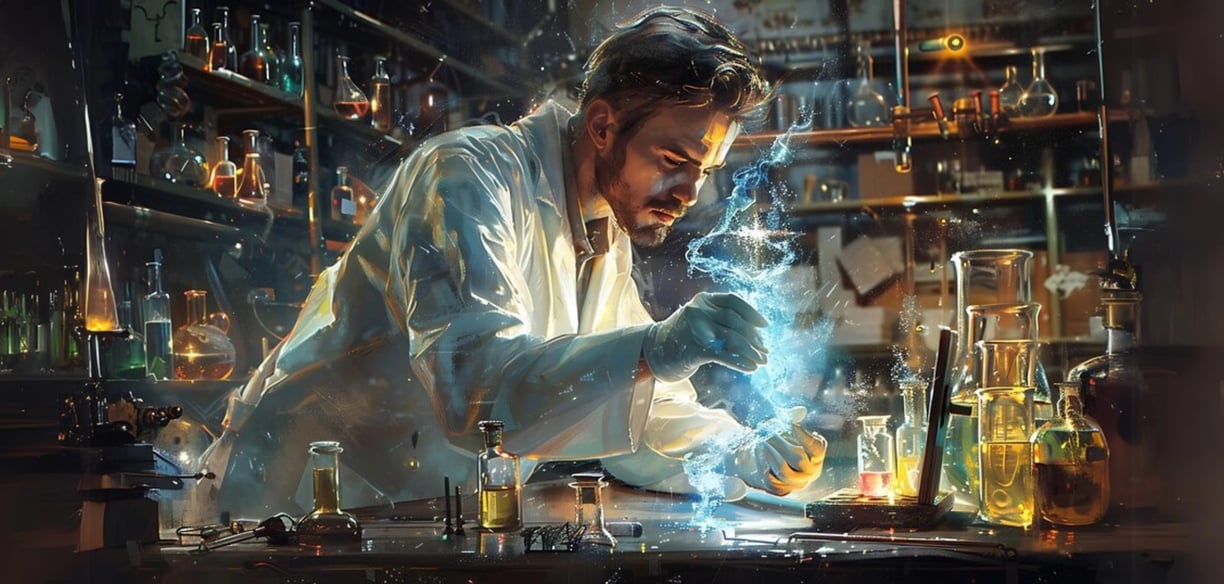
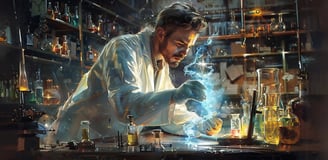
Person Griddying in a Science Lab AI
The AI has appeared as the revolutionary factor for many previous years affecting the contemporary science laboratories, their operations, approaches to the analysis of data, and their application. The Meishu “person griddying in a science lab AI” idea is the best example of the interaction between basic human ingenuity and state of the art AI capabilities. This collaboration is revolutionising scientific discovery as researchers are able to map large data sets, do high-level simulations and make history in this field.
The Evolution of AI in Science Laboratories
The incorporation of AI in science labs started as soon as machine learning and data analytics emerged. Earlier systems developed were not more complicated than mere sorting of data and recognition of certain patterns. Nonetheless, the availability of large language models and tools such AI/ML alters the applications of AI in laboratories. Today, AI systems are able to come up with hypotheses, intelligently code experiments, and perform review of scientific articles by other AI systems.
Also read >>> https://lyrifii.com/busted-smith-county/
Applications of AI in Experimental Research
AI is used in various forms of experimental research and is applied in chemistry, biology, and physics fields. For example, in drug discovery, AI learns hundreds of thousands of compounds, and then it selects the potential drug discovery ones. These systems can predict molecular interactions within extremely high levels of accuracy and so they displaced the time and costs of other methods.
In chemistry, AI helps to fine-tune such reactions like Sonogashira coupling which is very important in sonogashira coupling organic synthesis. AI tools mimic reaction pathways and determine yields, so chemists know how to optimise them for the highest efficiency. In the same manner, computational simulations driven by artificial intelligence enable researchers to perform hypothetical experiments all extra in silico.
Enhancing Precision in Pathology
AI has disrupted the field of pathology to improve the likelihood and timeliness of particular tissue tests. Built on annotated datasets, machine learning algorithms define patterns suitable for differentiation in biopsies which are not visible by naked eye. This capability holds distinct potential in the present day diagnosis of some diseases such as cancer that if diagnosed at and early stage can be cured.
Besides that, AI systems help reduce the risk of tissue contamination as they autonomously perform sample handling tasks. Robotic system and AI coordination provide sterility and repetitiveness, which increases when using machines instead of humans.
Virtual Labs: A New Era of Learning and Research
AI is making scientific aids and information accessible by using virtual laboratories. These platforms mimic the laboratory setting by offering the user a real world environment where he/she is confined to experimentation. Virtual labs are even more important during students training as they can familiarize with the lab equipment as well as experimental procedures.
Northwestern University’s virtual lab initiatives are an instance of this trend. Following the principles of artificial intelligence, the pedagogical process involves imitation of certain phenomena that allow explaining some theories or identifying specific skills in students. These virtual environment are also essential in tackling factors such as restricted access to lab facilities and issues to do with environmental conservation.
AI and Climate Change Research
Innovations in AI are important to solving grand challenges such as climate change. Today’s AI also learns climate changes and measure human impact on the environment as it deals with large sets of environmental data. Such knowledge is useful in making policies and strategies of addressing the impacts of climate change.
Also, sustainable technologies are promoted by AI tools. For example, the machine learning enhances the efficiency and capacity of renewable energy system designs. Self-propelled advancements in material science are also useful in developing sustainable solutions for usually used materials.
The Role of AI in Big Data Analysis
Big Data exists because scientific research involves creating vast amounts of data in doing studies. AI performs very well in making sense out of such data sets as become a rich source of new trends and correlation patterns for the researchers. AI/ML models deal with complicated data formats, including genomic sequences and chemical database, than any human being in any existent world.
As for bioinformatics, an application of artificial intelligence identifies genetic patterns within genome sequences that are associated with diseases. That is why the current studies introduce the possibility of creating a concept of a personalized medicine that is adjusted to the genetic plan. Likewise, in environmental science, AI devices used in estimating the extent of deforestation and shifted trends of urbanization from satellite images.
Also read >>> https://creatify.click/paradowski-ai-are-you-ready
Challenges and Limitations of AI in Science Labs
However, the use of AI in science labs has its problems. Such over-fitting in machineries is dangerous in machine learning and can significantly give wrong predictions thus effecting research results. To address this problem, it suffices to perform a proper selection of training data and apply stringent validation procedures.
The next issue is concerned with the ethical issue of AI development and its usage. Issues of data protection, algorithm, and decision making biases, as well as the question of who is held responsible for the application of AI in science have to be responded to. Cooperation with human scientists and AI systems in that sense is necessary to address these issues and bring the effectively of AI to their full potential.
Future Prospects of AI in Science Labs
AI holds a greatly anticipated role in the future of scientific laboratories based on continuous improvement of technologies linked to artificial intelligence. Neural networks including generative adversarial networks (GANs) of fields of experiment designing and data analytical systems are expected to emerge significantly soon. Year on year, lab management is set to become more and more automated by advanced AI systems which will enhance the effectiveness of any research institution by allowing the researchers themselves to focus more on finding innovative solutions to problems.
AI applications in drug development can be said to be most effective. Using Artificial Intelligence at the molecular level, it makes the process of discovering new diseases like antibiotic-resistant drugs faster. Solutions have the capability to prevent an estimated number of millions and alter healthcare systems worldwide.
FAQs
What does "person griddying in a science lab AI" mean?
Refers to the process of interaction between human researchers and Artificial Intelligence systems in structuring, processing, and utilising the scientific data sourced across particular laboratories. As a result, ‘griddying’ refers to processes of organizing and making sense of a large amount of data in a regular pattern.
How is AI used in drug discovery?
AI uses its algorithms to search chemical compound databases for compounds that are likely to be utilizable as drugs, estimate how these compounds will interact with others, and define parameters for experimentation. This helps to reduce time of identifying drugs and at the same time minimize costs and risks.
What are virtual labs, and how do they work?
Virtual labs can be referred to as special AI-driven open platforms that mimic real laboratories. Their use offers touch screens whereby experiments can be performed and data analyzed, and this makes it easier to learn science.
What challenges do AI systems face in science labs?
Some of the main challenges experienced by AI systems include over fitting, ethic concerns, and requirements of high quality data for training. These problems can be solved by the cooperation of different practices and AI developing all the time improvement.
How does AI contribute to climate change research?
AI models process information on different climate for the purpose of understanding the effects of human activities and designing technologies for sustainable living. These insights help in making decisions regarding dealing with climate change.
What is the future of AI in science labs?
The future also comprises better AI technologies such as neural networks and GANs to expand the automating process of laboratories, analysis data and speed up the discoveries in the science field.