AI Infrastructure and Operations Fundamentals: Drive Wins
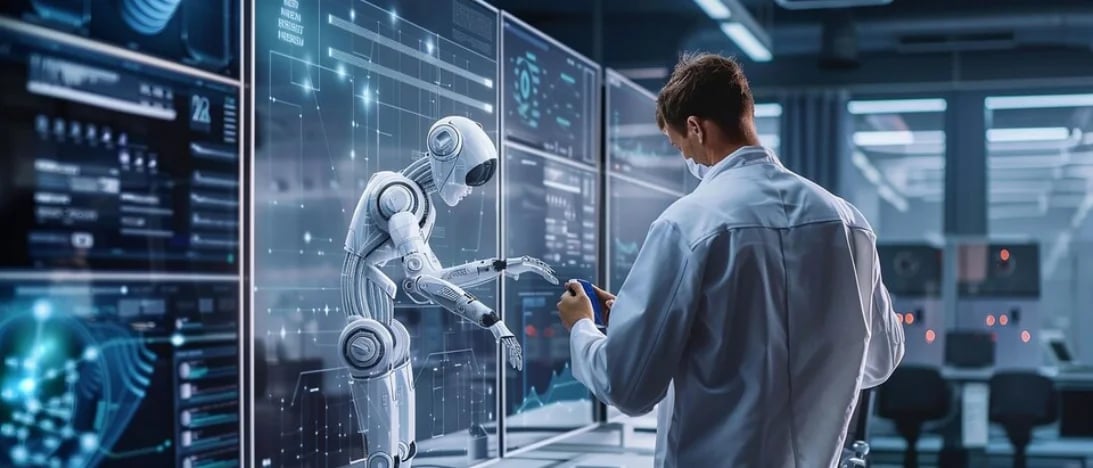
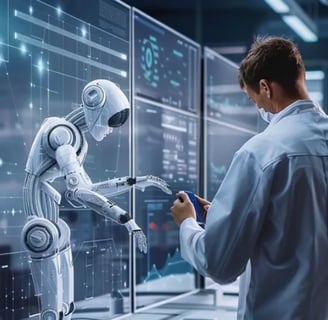
Introduction
Artificial intelligence (AI) has rapidly evolved, becoming a vital component of modern business and technological landscapes. AI infrastructure and operations provide the backbone for AI systems, from machine learning models to natural language processing. These components power innovations in healthcare, finance, autonomous vehicles, and more. By understanding AI infrastructure and operations fundamentals, businesses can unlock their full potential in data-driven decision-making and efficiency.
Understanding AI Infrastructure
Key Components of AI Infrastructure
AI infrastructure encompasses hardware, software, and data management resources critical for processing complex models and algorithms. Essential components include powerful GPUs (graphics processing units), high-speed storage solutions, and reliable data pipelines. With an infrastructure designed to support massive computational requirements, AI models operate more efficiently, significantly boosting performance and reducing lag times.
Role of GPUs and TPUs in AI Infrastructure
Graphics processing units (GPUs) and tensor processing units (TPUs) are the powerhouses behind AI computations. GPUs, initially built for gaming, are exceptionally effective in handling the parallel processing needs of machine learning algorithms. TPUs, engineered by companies like NVIDIA and Google, enhance deep learning processes by supporting faster computations, enabling AI models to learn and adapt in real-time.
NVIDIA Solutions and AI Infrastructure Evolution
NVIDIA is at the forefront of AI infrastructure innovation, providing solutions that include GPUs designed for AI applications and comprehensive software frameworks. NVIDIA’s CUDA framework, for example, empowers developers with tools for building AI models on GPU-accelerated platforms. With solutions like NVLink, NVIDIA makes it possible for data centers to process and share massive datasets, enhancing AI operations.
Also read >>> https://creatify.click/ai-chatbots-for-ecommerce
AI Operations Fundamentals
Overview of AI Operations and Its Necessity
AI operations (AIOps) leverage machine learning to optimize IT operations, helping organizations manage large-scale data environments. AIOps enable predictive analytics, which can forecast infrastructure needs and automate decision-making processes, reducing human error and improving operational efficiency.
Integrating AIOps for Improved Efficiency
Incorporating AIOps into traditional IT operations can enhance performance monitoring, problem detection, and system maintenance. Through predictive maintenance and real-time insights, AIOps help businesses reduce downtime and optimize resource usage. As a result, companies benefit from smoother operations and cost savings, particularly within data-intensive environments.
Core Concepts in AI Infrastructure
Infrastructure and Data Management for AI
Data management plays a central role in AI infrastructure, as data is the lifeblood of any AI model. Effective data management involves robust storage solutions, data cleansing, and secure data transfer systems. By ensuring data is organized and accessible, businesses can streamline AI model training and enhance predictive accuracy.
Training and Model Development Essentials
Training AI models is resource-intensive, often requiring sophisticated algorithms and extensive computational power. Model development involves several stages, including data preprocessing, model selection, training, and evaluation. High-performing infrastructures that support rapid data processing allow teams to train models faster and deploy them at scale.
Security and Compliance in AI Operations
AI infrastructure and operations are vulnerable to security risks. Protecting data privacy, complying with regulatory standards, and implementing strong cybersecurity protocols are paramount. Compliance standards, such as the General Data Protection Regulation (GDPR), require businesses to maintain strict control over data usage, reinforcing the need for security in AI systems.
AI Models and Systems
Types of AI Models (Deep Learning, NLP, etc.)
AI models come in various forms, including deep learning models, natural language processing (NLP) systems, and recommendation algorithms. Each type has unique infrastructure needs and benefits specific applications. For example, NLP models are widely used in chatbots, while deep learning models support image recognition and self-driving cars.
Model Training and Deployment Best Practices
Efficiently deploying AI models demands robust infrastructure to support continuous updates and scalability. By following best practices, such as leveraging hybrid cloud solutions, businesses can ensure models remain up-to-date without overloading their infrastructure.
Popular AI Certifications and Courses
Courses from Leading Institutions (MIT, UC Berkeley, IBM)
Reputable institutions, such as MIT, UC Berkeley, and IBM, offer AI courses covering infrastructure fundamentals, machine learning, and data science. These courses often include hands-on training and capstone projects, providing foundational knowledge and practical experience.
NVIDIA-Certified AI Programs
NVIDIA offers certification programs that focus on deep learning, neural networks, and GPU computing. These certifications, recognized globally, validate expertise in handling AI infrastructure and applications, making them ideal for professionals in data science and machine learning.
Also read >>> https://lyrifii.com/discreettilly42-from-maine/
Practical Applications of AI Infrastructure
Hybrid Cloud and AI Operations
Hybrid cloud solutions provide scalable, flexible platforms that allow businesses to deploy AI models across multiple environments. This setup enhances data access and model deployment, giving businesses agility in handling large datasets and diverse applications.
AI in Data Centers and Enterprise Solutions
Data centers are integral to AI operations, enabling large-scale data processing and model training. By investing in AI-focused data center solutions, enterprises can streamline their operations and support their AI models' performance needs.
Advanced AI Operations Concepts
AI in Real-Time Operations
Real-time AI operations enable instant insights and decision-making capabilities, vital for industries such as finance and healthcare. Through live data processing, AI systems can respond to changes dynamically, helping organizations stay competitive and agile.
The Role of Large Language Models and Generative AI
Large language models, such as those used in generative AI, allow machines to understand and generate human language. These models have practical applications in chatbots, content generation, and language translation, advancing how businesses interact with technology.
Building a Career in AI Operations
Skills Needed for a Career in AI Infrastructure and Operations
A career in AI infrastructure demands skills in data management, programming, and machine learning. Professionals also benefit from hands-on experience in AI operations, provided by internships, training programs, and real-world projects.
Hands-On Experience and Capstone Projects
Practical experience is essential for mastering AI infrastructure. Many courses include capstone projects that allow learners to apply theoretical knowledge to real-world scenarios, further developing their expertise.
AI Security and Compliance
Essential Security Measures in AI Systems
Securing AI systems requires layered security protocols, including encryption, access control, and regular audits. Implementing these measures helps prevent data breaches and ensures compliance with data protection standards.
Compliance Standards and Risk Management
Compliance standards, such as GDPR, mandate strict protocols for data management. By adhering to these standards, businesses mitigate risks associated with data privacy and safeguard their AI infrastructure from potential vulnerabilities.
Future of AI Infrastructure and Operations
Trends in AI Development and Infrastructure Evolution
The future of AI infrastructure lies in advancements such as quantum computing, edge AI, and improved data processing frameworks. Companies like NVIDIA lead the way, continually innovating to meet the needs of a rapidly growing AI landscape.
FAQs
What are the main components of AI infrastructure?
Key components include GPUs, data storage solutions, and data pipelines that support efficient processing and management of AI models.
How does AI operations improve business efficiency?
AI operations streamline processes, automate decision-making, and reduce human error, leading to better resource management and cost savings.
What are some trusted certifications for AI operations?
Certifications from institutions like MIT, UC Berkeley, IBM, and NVIDIA validate expertise in AI infrastructure and operations.
How can businesses secure their AI infrastructure?
Implementing strong encryption, access controls, and regular audits ensures data protection and regulatory compliance.
What are the future trends in AI infrastructure?
Trends include advancements in quantum computing, edge AI, and cloud-based solutions that promise to enhance AI performance and scalability.